Hyper-SD LoRAs: Trajectory Segmented Destillation for Better and Faster Outputs in FLUX.1/SDXL/SD Models
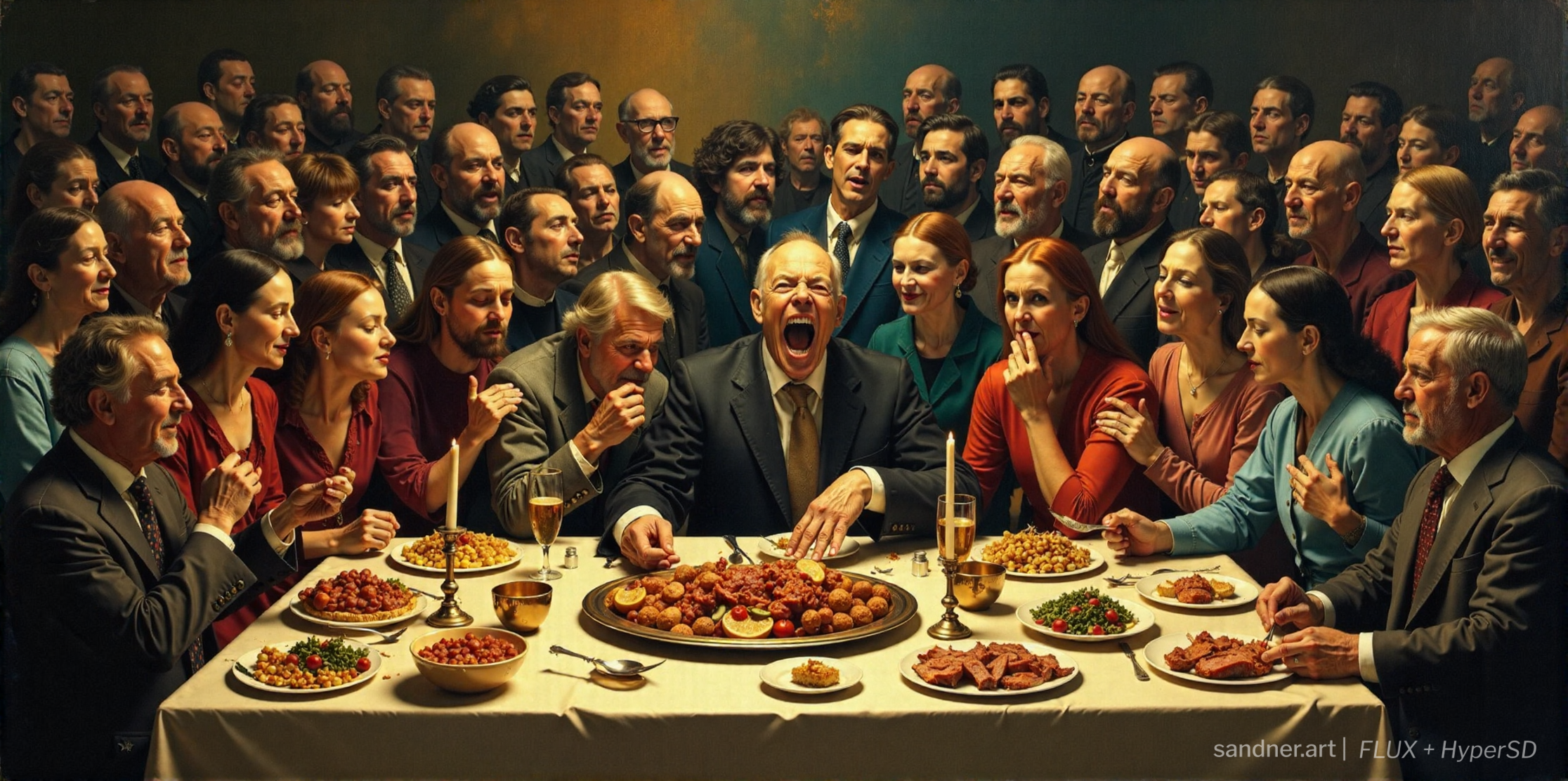
Hyper-SD offers an advantage in Stable Diffusion by allowing FLUX users to reduce the number of generation steps while maintaining or even improving image quality. This technique is applicable to various Stable Diffusion models, including SDXL, SD3, and SD 1.5. By optimizing the generation process, Hyper-SD provides a faster and more efficient way to create high-quality images.
Using Hyper-SD LoRAs
Download
- Original https://huggingface.co/ByteDance/Hyper-SD/tree/main
- FP16 (half-size) version of Hyper-FLUX.1-dev-8steps-lora https://huggingface.co/nakodanei/Hyper-FLUX.1-dev-8steps-lora-fp16/tree/main
SD/XL Note: Use CFG Scale 1-1.5 ('CFG-lora' versions require 3-7 CFG).
A1111/Forge
Add Hyper-SD LoRA as a LoRA to a prompt with a proper weight (guide on how to install Forge).
Weight for Hyper Flux is 0.125, Hyper SDXL around 0.75, Hyper SD15 around 1.
ComfyUI
1-step Unet checkpoint requires a specific scheduler node. LoRA versions are using standard sampler. Link to original comfyUI workflows and more information is in References. My test workflows on github. ComfyUI setup tutorial is here.
The LoRAs of Hyper-SD FLUX/SDXL/SD are compatible with ControlNet.
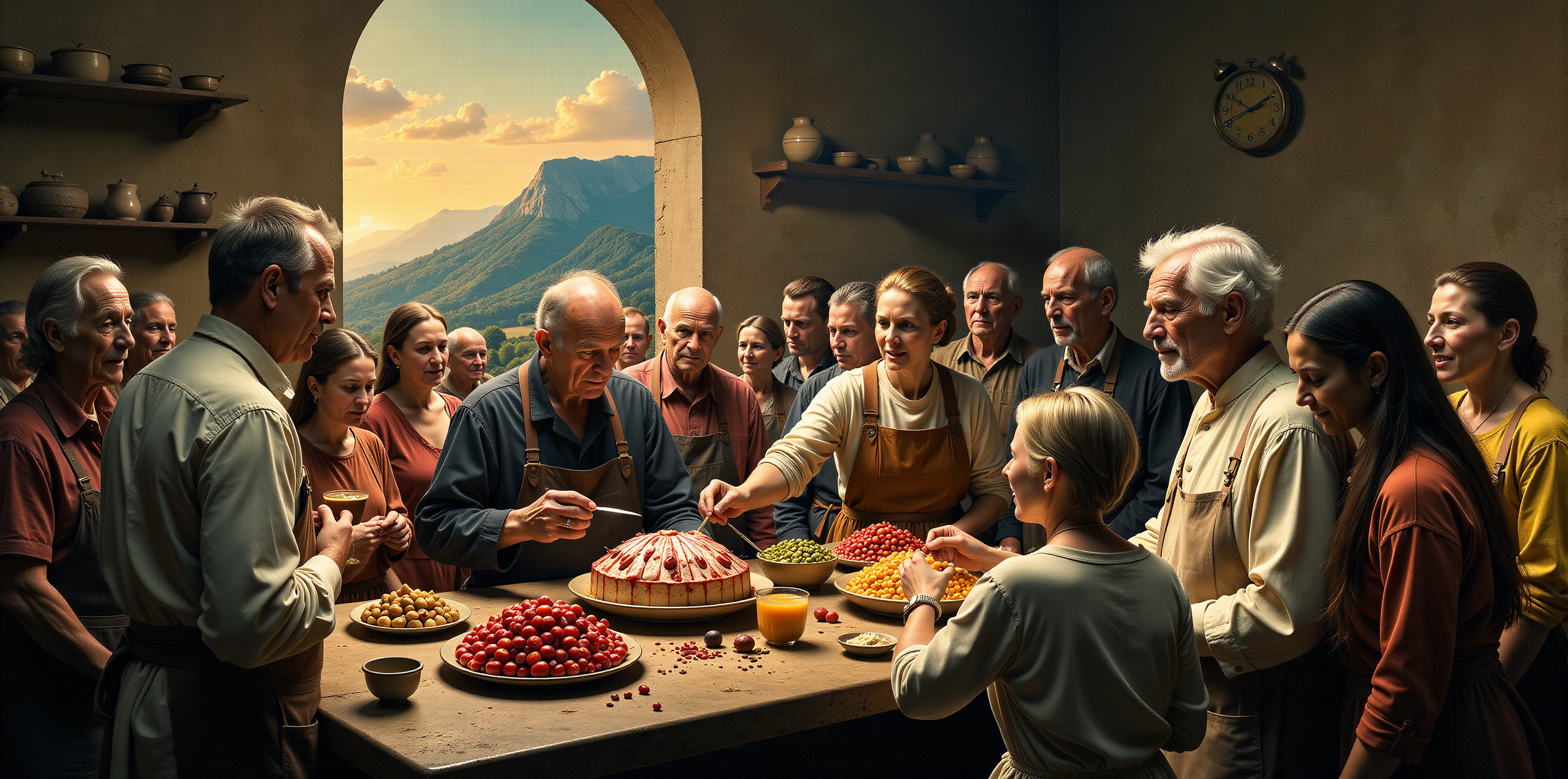
Results
The results obtained using Hyper-SD are surprisingly impressive across all three model ecosystems tested: FLUX, SDXL, and SD 1.5. While the SDXL Unet 1-step version exhibited some challenges in producing ugly noisy artifacts (and also produced more stylized results), these issues can be addressed through upscaling techniques and LoRAs. Hyper-SD (in LoRA forms) truly excels in rendering complex compositions with numerous figures, where it sometimes seems to surpass the limitations of the base models, particularly in terms of anatomical accuracy.
While speed might not be the primary concern when prioritizing image quality, the significant reduction in rendering time achieved with Hyper-SD becomes particularly valuable for FLUX (dev) models running locally, especially when using GPUs with limited VRAM. By eliminating approximately 5-10 steps per image, Hyper-SD can lead to substantial time savings.
FLUX (dev)
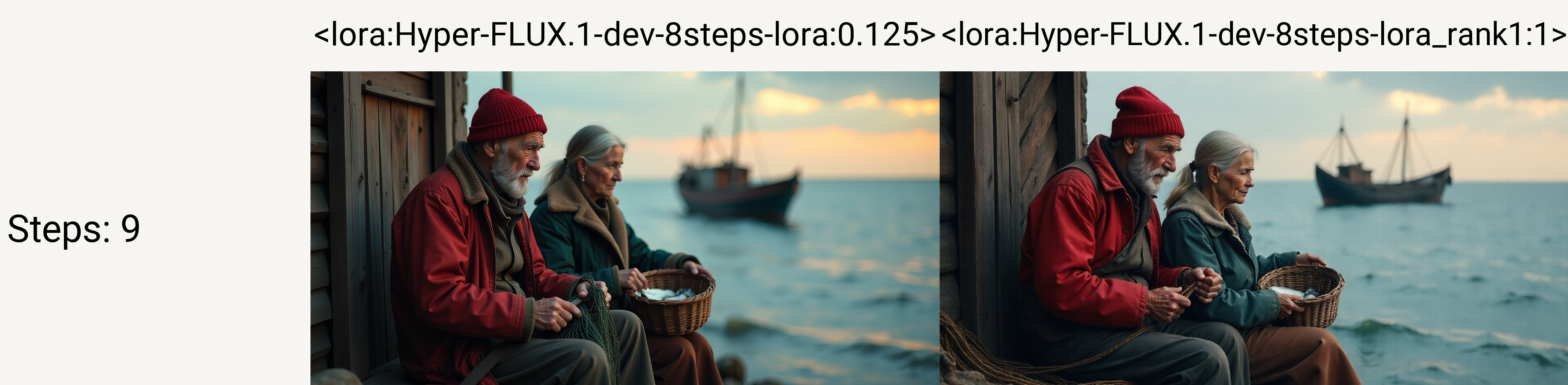
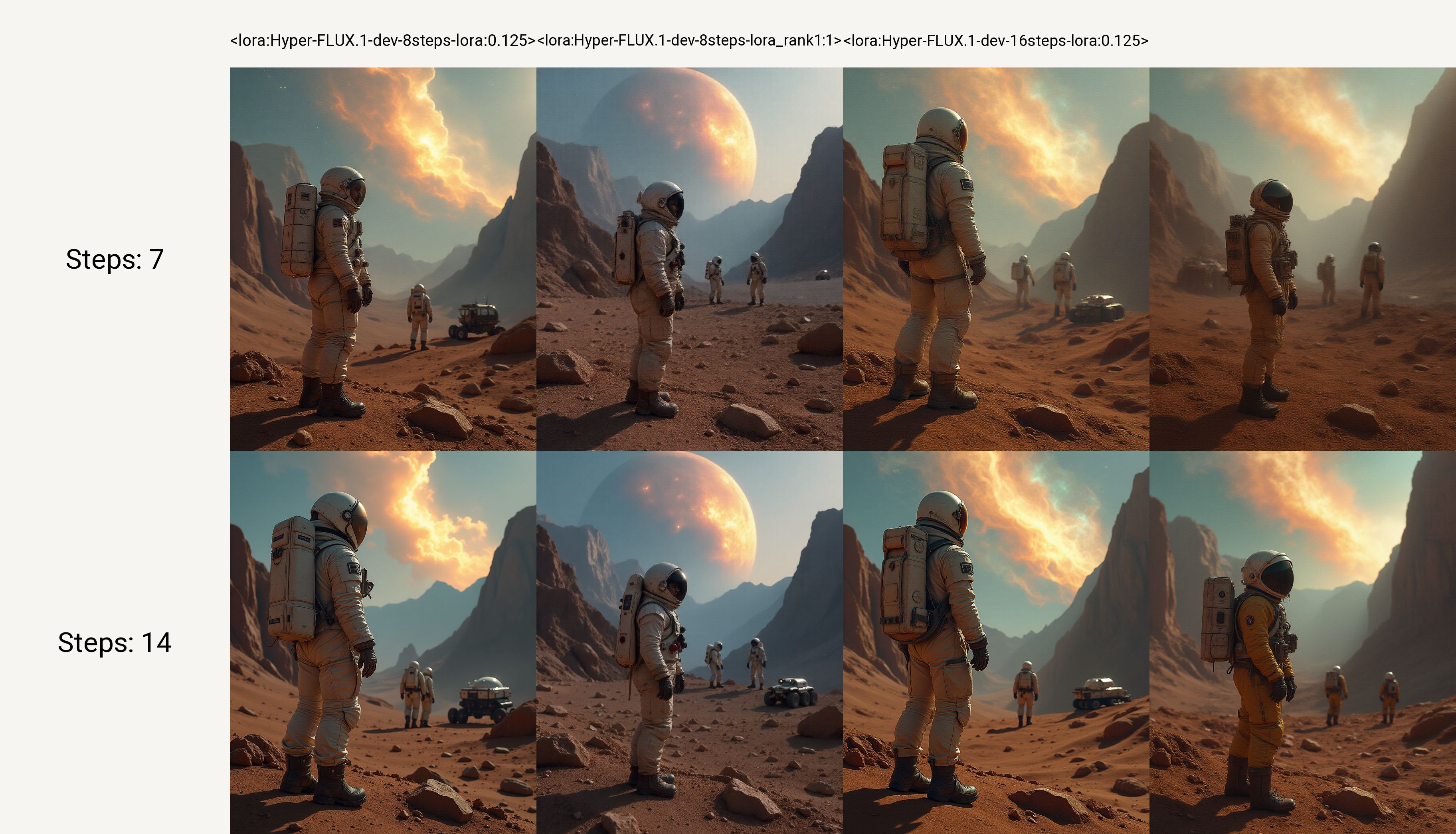
SDXL
Using Hyper-SD LoRAs may introduce some artifacting in 1-pass workflow.
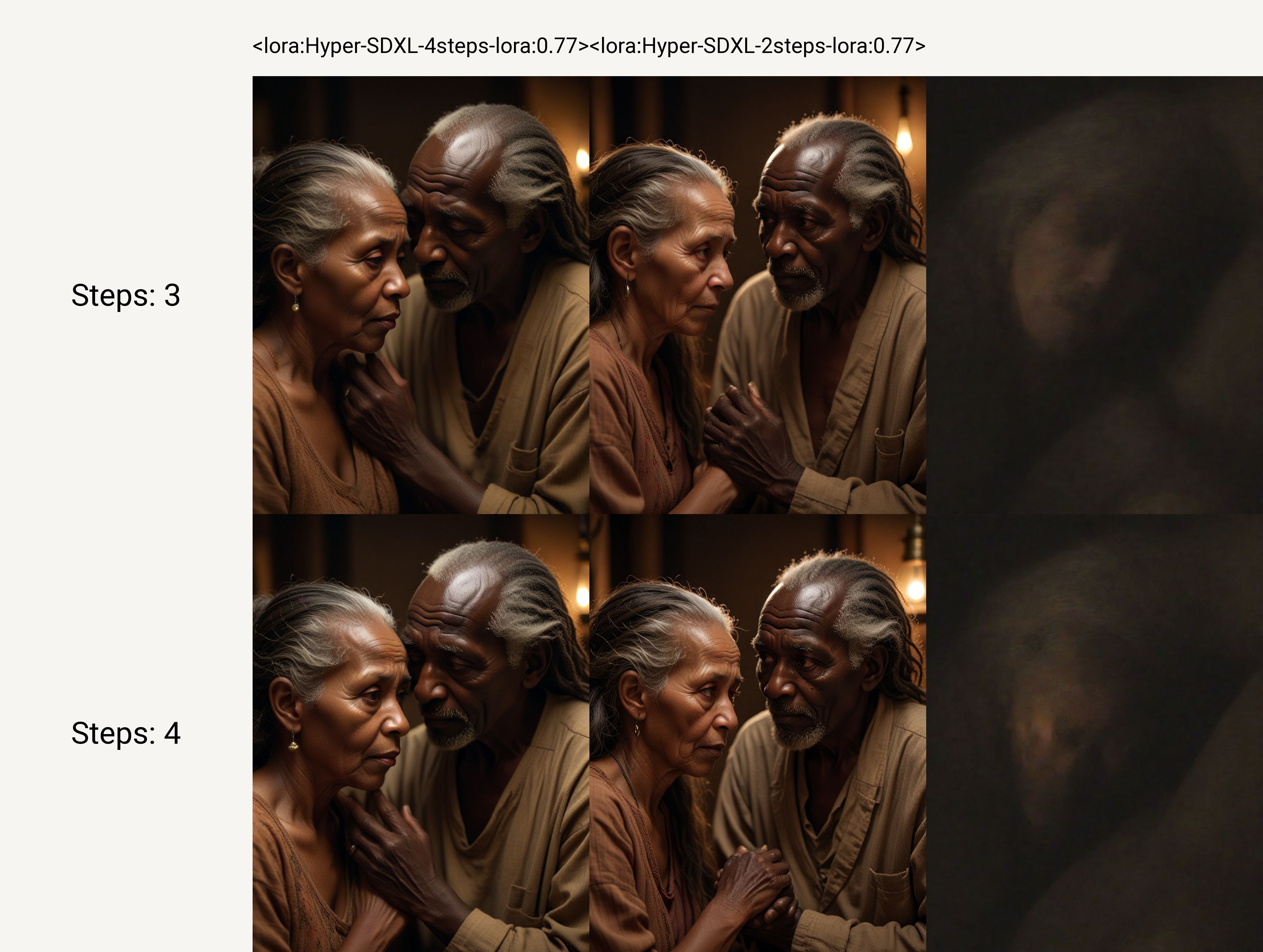
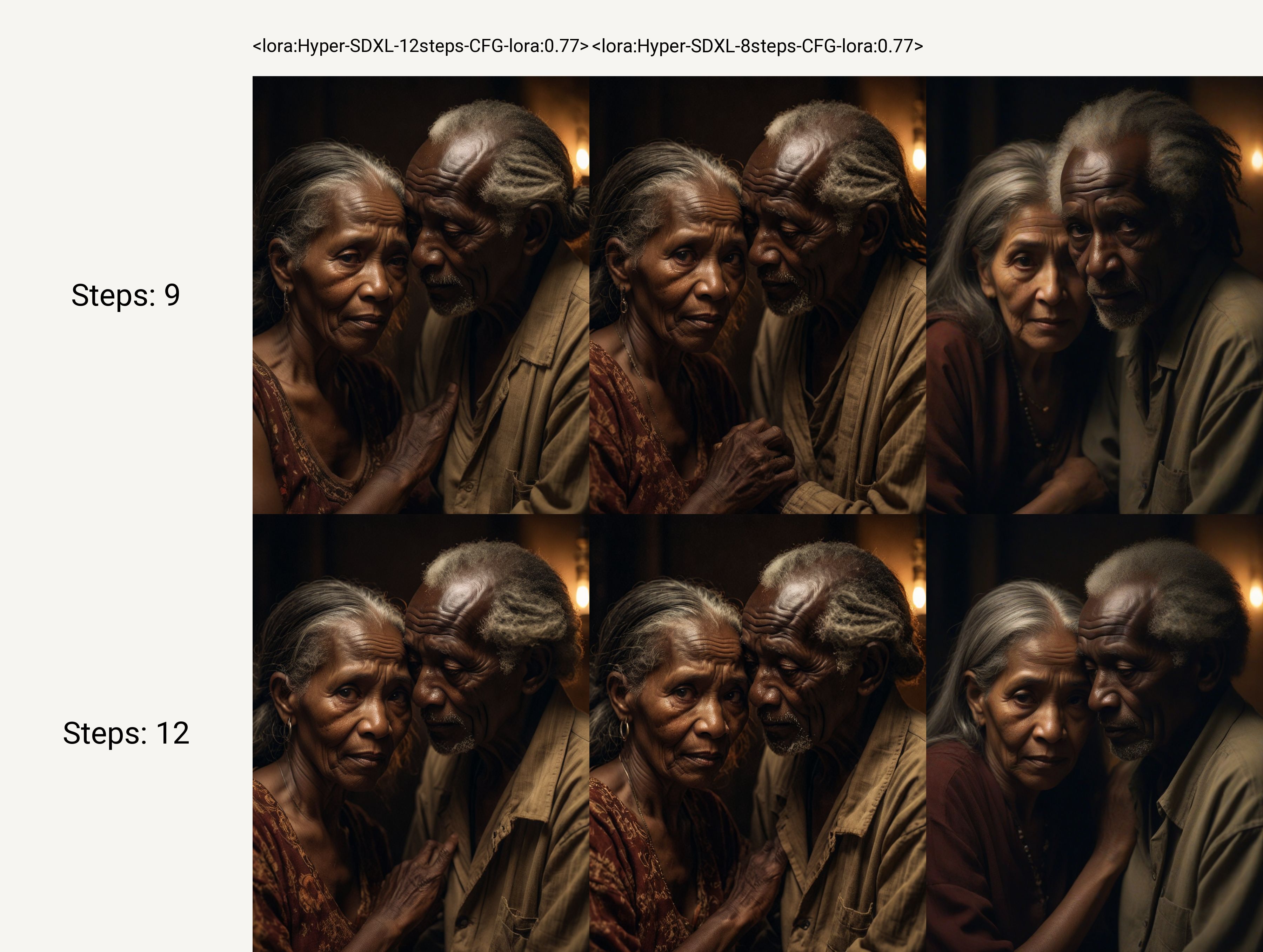
SD15
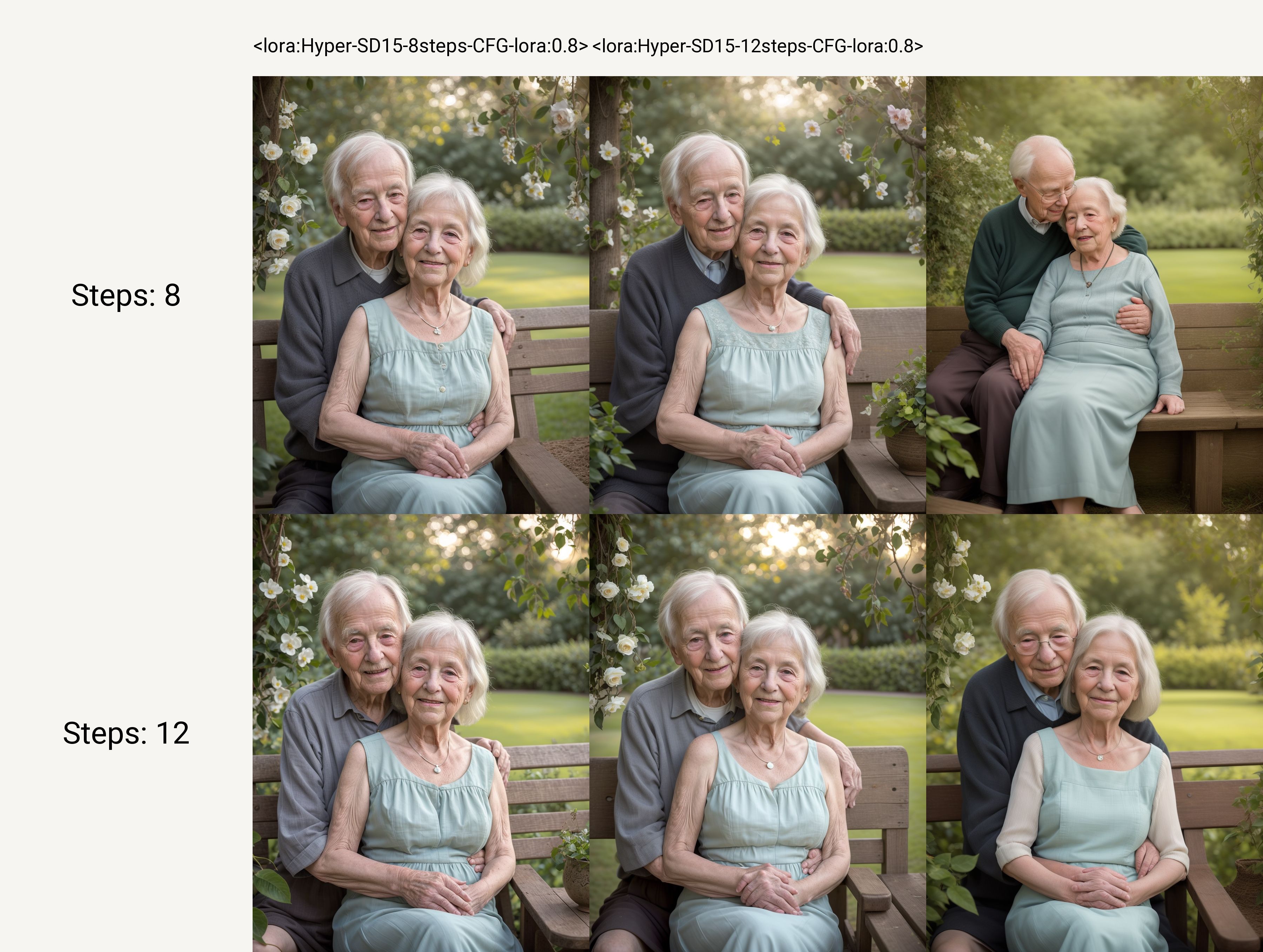
Unet Versions (SDXL)
- In Forge put 'Hyper-SDXL-1step-Unet-Comfyui.fp16' into models/Stable-diffusion, for generations use CFG = 1. It needs 2-3 steps to form image, may require 2-pass / Hires fix to generate a usable image.
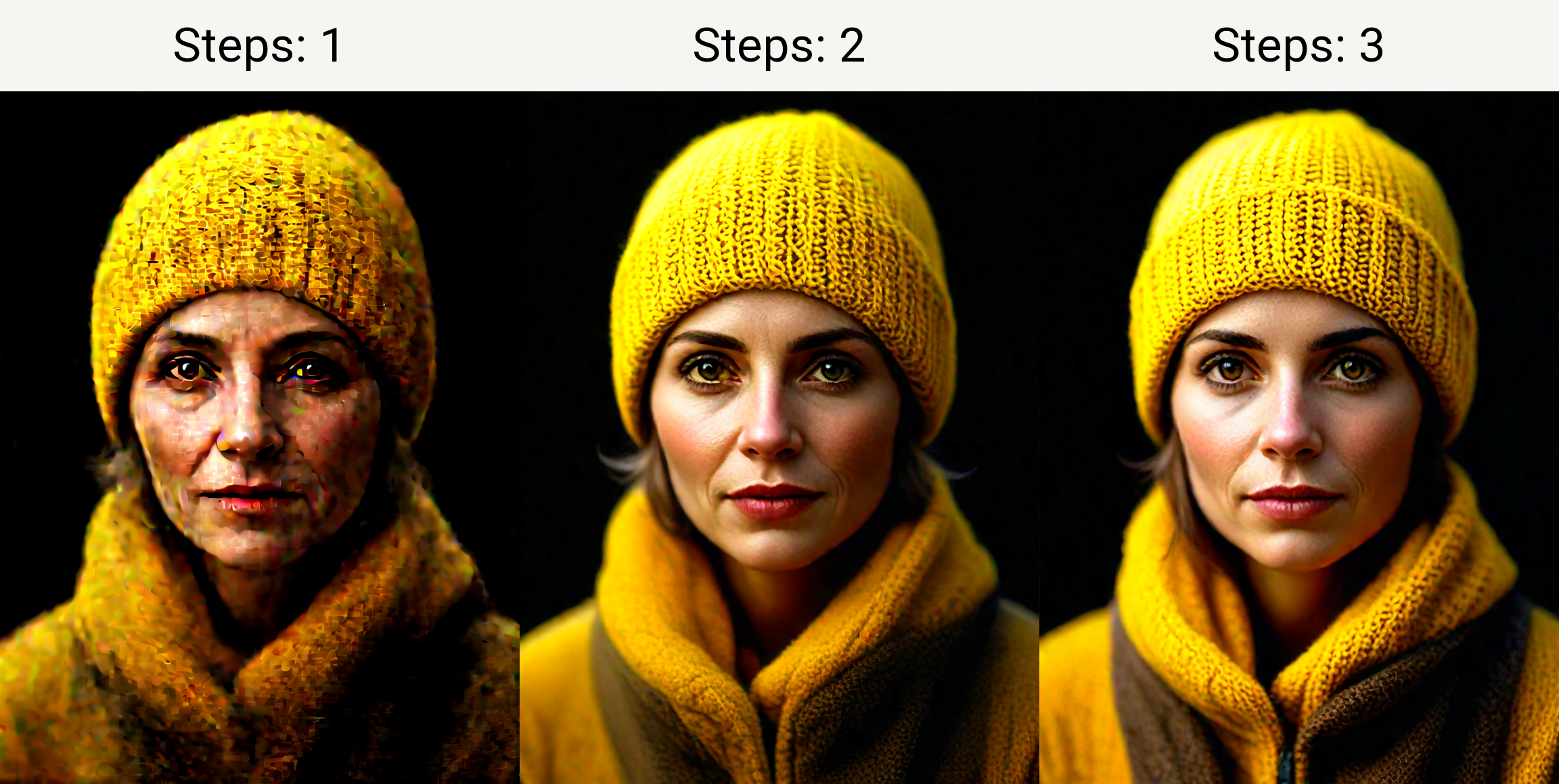
1-Step SDXL UNet for ComfyUI requires install of scheduler folder, see more details in References.
Alternatively, needed TCDModelSamplingDiscrete node (ComfyUITCD) is also installable via ComfyUI Manager (drag&drop HYPERXL-1stepUNET test images from my COMFYUI test workflows.
Conclusion
In conclusion, Hyper-SD LoRAs represent a nice addition to improve outputs of Stable Diffusion models. Overall, there are more benefits for FLUX dev model, where sparing some steps helps due to the slower generation— but for very low number of steps generations, FLUX (schnell) produces a better alternative (see also this comparison with Flux Turbo LoRA).
By optimizing the generation process through trajectory segmentation, this technique offers a compelling solution for achieving interesting image generation outputs. The benefits of Hyper-SD extend to various applications, even correcting outputs in some samplers/schedulers/models combinations—and being in a LoRA form it is also easy to use. Using very low number of steps produces artifacts in SDXL and requires more passes and upscaling techniques to remove them. It may be worth it for achieving interesting compositions—and with FLUX model it will help to get even better details.
References
- Paper Hyper-SD: Trajectory Segmented Consistency Model for Efficient Image Synthesis: https://hyper-sd.github.io/
- ComfyUI workflows https://huggingface.co/ByteDance/Hyper-SD/tree/main/comfyui, https://huggingface.co/ByteDance/Hyper-SD
- Test workflows https://github.com/sandner-art/ai-research/tree/main/HYPER-SD
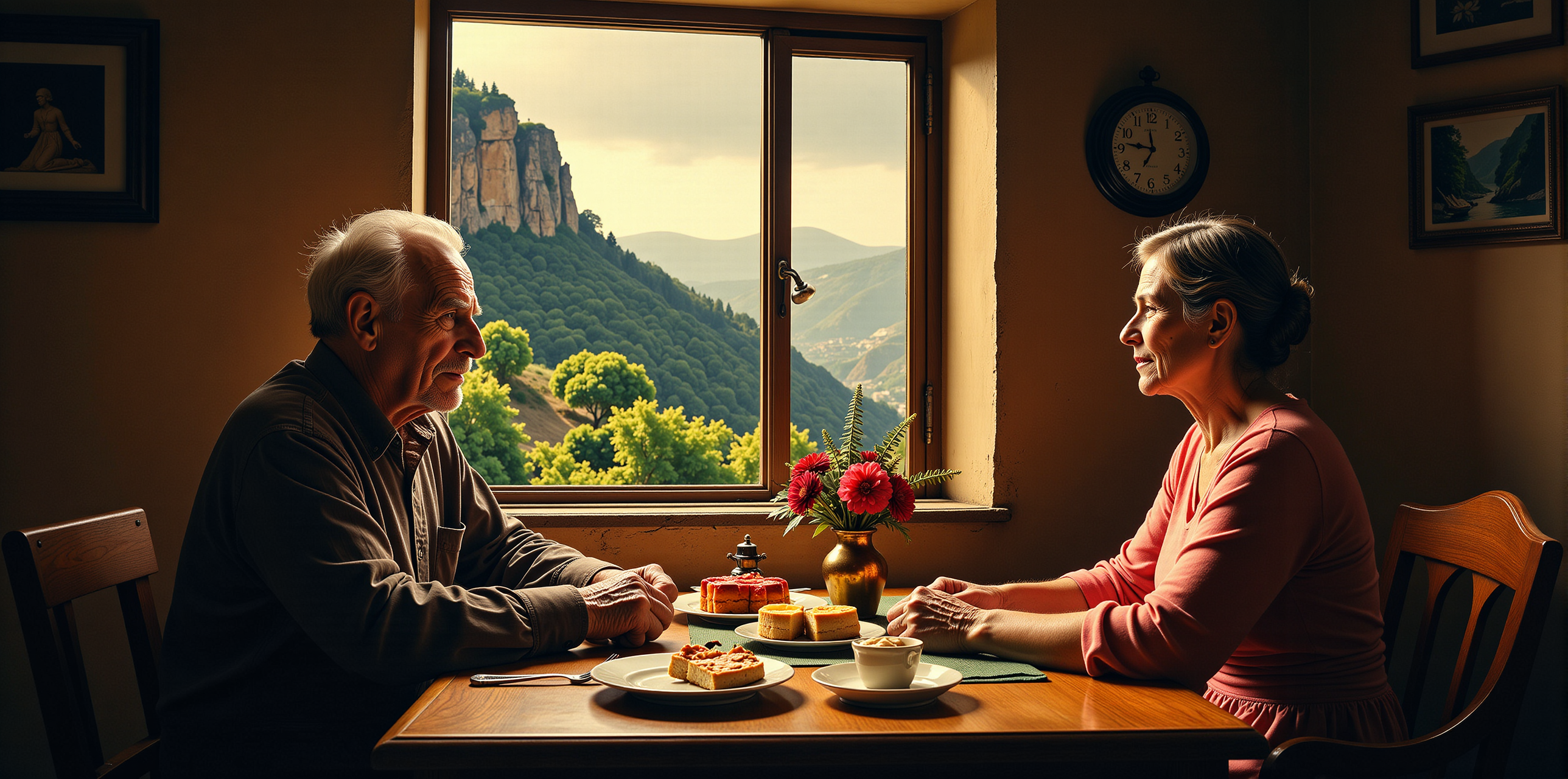